Have you heard? Generative AI (Gen-AI) is sweeping across the digital user research lands. Bunnyfoot consultants Louise Bloom and Mélissa Chaudet look at the good, the bad and the ugly of current uses.
Users
The most controversial aspect of generative AI use in UX research is replacing human users in testing. Services like syntheticusers.com promise to deliver authentic user insights through AI generated responses to test prompts.
Good?
Kwame Ferreira, the creator of Synthetic Users, believes that his service will ensure UX research gets done where it might otherwise be overlooked. A panel of AI generated-users deliver instant responses and removes the cost of recruitment and implementing testing with users IRL (In Real Life). There are also interesting options for training researchers with respondents when validity isn’t an issue.
Bad?
Like all AI, generated user insights are dependent on the information they are trained on. Where humans are unique and ‘trained’ on individualised experiences, generative AI responses have a common data set. It’s a bit like asking the same person to role play a type of person, it can only imagine what it already knows. Synthetic users can only imagine experiences based on learned best practices and other elements within an LLM (Large Language Model). They have no ‘lived experience’.
Ugly?
Synthetic users create a high risk of normalisation our view of a user experience. Machine learning models tend toward normative and ‘most likely’ representations. Ferriera believes his user insights should have ‘the same biases we do’, but for many UX researchers the goal is to move beyond our bias by amplifying the experiences of those who have unique, less represented and diverse needs.
Prototyping
Pre-launch and experimental user research relies on a ‘prototype’. These can range from paper representations to fully developed digital products that are yet to be made public. Transformative AI, can take an input in one format and transform it into another type of output. Services like Uizard (https://app.uizard.io/) are using this technology to rapidly generate testable user interface designs from text prompts, screenshots etc., bypassing the need for specialists in user interface (UI) design or coding.

Good?
Transformative Gen-AI lowers the skills needed to create high-fidelity, clickable prototypes. Rapid, iterative testing with end users, and near-instant modification, lowers the cost of testing and speeds up the UCD lifecycle.
Bad?
Generative AI tempts us to go straight to high-fidelity prototypes, when this isn’t always the best way to collect feedback. For example, in early-phase testing, users’ feedback on colour, copy and imagery etc. can stop us from understanding how they feel about the concept in general.
Ugly?
As we cut out the craft of generating bespoke designs, will we arrive at a future full of generic designs, lacking originality and any unique qualities? We could create a homogenous landscape of digital interfaces where it’s hard to make your product stand out.
Content
Generated content is the area where AI has been most used to date. Testing prototypes have always used dummy text and images as placeholders, such as ‘lorem ipsum’ and stock imagery. Generative AI can rapidly create meaningful content in line with a range of parameters, using simple natural language prompts.

Good?
Again, Gen-AI removes the barriers to creating testable UIs. The tools are fast and easy to use, and can respond to a range of different needs, including character count, style, language and reading level. Images are increasingly realistic and can be quickly changed and replaced.
Bad?
Maybe one of the biggest risks of using Generative AI content is that you need to check it carefully. Without careful prompting, AI can produce incorrect and even offensive copy and images and be particularly clumsy when using different tones of voice.
Ugly?
Bias is inherent to AI and has the potential to harm individuals and society until we are able to mitigate those risks. As researchers and designers, we can support the process of minimising bias by ensuring diverse voices are represented.
Test protocols
AI research design is also already with us. As best practice emerges around test scripts, questioning styles, surveys and usability test prompts, can the machines do the work of talking to the people?

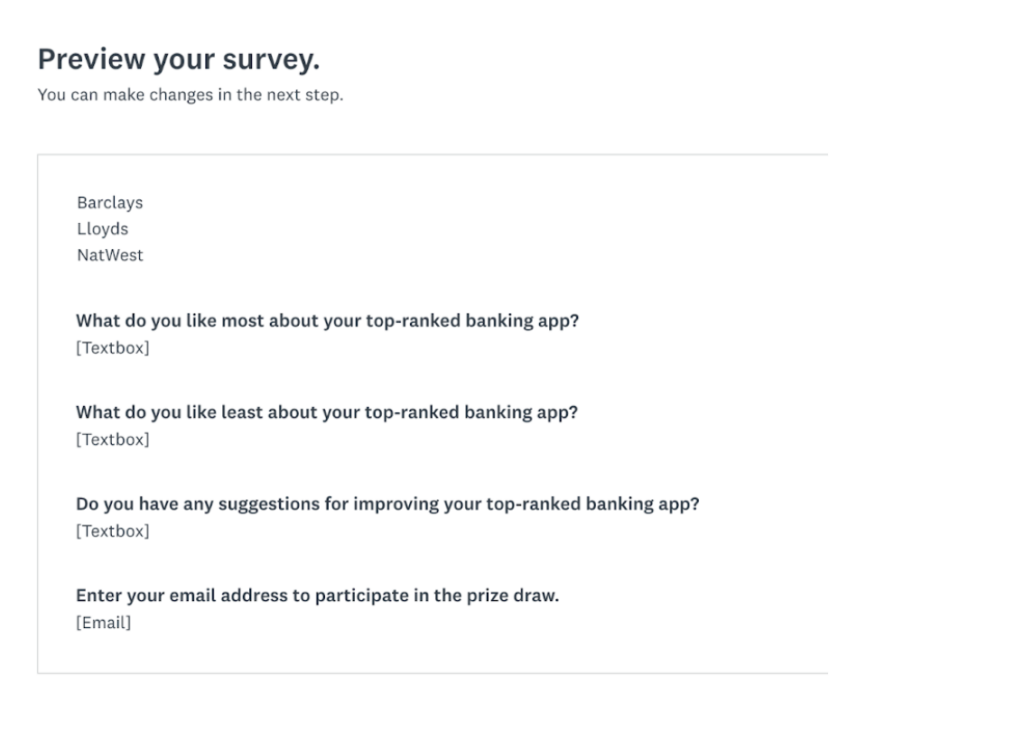
Good?
When prompted correctly, and provided sufficient input, AI is a great way to create a first draft of a test protocol. It can support with rephrasing questions and suggesting additional prompts. For entry-practitioners in particular, AI support is a quick way to level up.
Bad?
For complex scenarios, or sensitive topics, the need for experienced professionals with understanding of how to manage question design for engagement and cognitive persuasion etc. is critical. We talk more about this in our UX Training course on user and customer research methods.
Ugly?
AI is so quick that there is a temptation to bypass the pre-thinking that goes into writing a test protocol to speed up the process. This can lead to conducting research that fails to meet the key research objectives or to provide quality user insights. For example, AI often falls short in areas such as rapport-building questions, questions flow logic, participant engagement and cognitive persuasion. Practitioners should be especially careful when creating test protocols for complex or emotionally sensitive topics.
Analysis
Affinity mapping, sentiment analysis, thematic derivation, we’ve been using AI to augment our analytics for some time now, even outside of quantitative data sets. Gen-AI as a digital assistant can review our transcripts, notes and analyses to help us interpret qualitative data and identify key evidence.

Good?
As a research assistant, generative AI has enormous potential to be a partner and derive patterns and high level insights from transcripts following test sessions. It can also validate researcher’s findings against the data collected, and identify oversights and omissions.
Bad?
People’s statements often carry complex meaning and nuances. Tone of voice can convey emotions like sarcasm or satisfaction, but AI still struggles to detect these nuances. We can train AI to understand and reflect issues like severity of usability issues according to common best practice, but the realities of human impacts are complex. AI outputs are essentially quantitative analyses of data and patterns, as humans we are better at detecting outliers that matter.
Ugly?
Generative AI could come to replace human thought in reviewing research data and generating insights. This is a risk of two fronts. The speed that insights can be generated will leave little room for review and reflection, as product teams are under pressure to deliver in very short time frames. Product owners will have to trust that the insights provided by AI are robust and valid, despite known issues with AI-analysis of human behaviours.
Reporting
Surely, generating UX reports is an area where generative AI is best placed to deliver value and improve outcomes. In the Nielsen Norman Group article on AI as a UX Assistant, writing research reports using Chat-GPT and plugins to create first drafts is one example.
Good?
Gen-AI can really help to level the playing field for people who don’t enjoy or feel skilled in writing. Rewording text and suggesting first drafts is a common use of AI. Generative AI can also assist with organising report content into clear sections, reducing the challenge of structuring the material.
Bad?
As Gen-AI still needs a heavy review and complex prompting to get things right, creating insightful and comprehensive research reports can take just as long with an AI assistant.
Ugly?
If the machines do the work of critical thinking and communicating findings, we are looking at a future workforce who have not had opportunity to develop these skills. Research reports are the mechanism by which data influences design and future thinking. By removing people from the reporting process, we miss the last opportunity for people to safeguard against issues arising from unchecked AI involvement.
Should the machines do the work?
AI-Generation is already here and teams that are using it in their workflows can speed up and deliver higher quality outputs with less human input, UX experience or research skills. Research has been seen by technologists as an obstacle to getting products to market at times, and UX has long struggled with the best way to integrate with Agile design and development.
Transformative natural language prompting (NLP) lowers the barrier to entry and means that more people can get involved in iterative research practices.
LLMs and the other data sets that drive the outputs of AI-generation engines are limited, relying on data and learning that is subject to inherent bias and narrow-sightedness. Humans have bias and limitations too, but are better able to self reflect and extend their experience.
In the long term, AI-led processes could erode the knowledge and skills base of our community. In each step of the UCD/UCR process, AI-generated output is reasonably helpful when qualified by a human expert; in combination there is potential for user experiences and interfaces that have been researched, designed, tested and delivered without human input, to the detriment of inclusive design and creativity. As practitioners we have a significant role to play in shaping this new landscape toward positive outcomes.
A hybrid world
Learning to leverage AI to support rapid UX research and delivery will enhance your UX processes when combined with the skills and experience of human UX practitioners to review and guide it. At Bunnyfoot, we have years of experience training practitioners in the kind of foundational skills and principles that will help your team ensure that you remain in control and deliver quality, compassion and joy in your digital products.
See our list of professional UX training courses and contact Jo Hutton to learn more.
